Japan—Researchers have automated a complex and labor-intensive process for analyzing the results of X-ray diffraction studies, which are used to determine the structure of crystalline materials. The team of the National Institute for Materials Science (NIMS) has described the development and application of their technique in the journal Science and Technology of Advanced Materials: Methods.
X-rays fired at a crystal interact with the geometric arrangement of its particles and are diffracted in many directions in a complex pattern of rays that depends on the crystal’s precise structure. Experts analyze the pattern and intensity of the diffracted X-rays to determine the crystal’s internal arrangement. This is a powerful and widely used process for revealing the three-dimensional atomic structure of new materials.
A well-established mathematical procedure, called Rietveld analysis, is used for interpreting X-ray diffraction data, but it is time-consuming and requires manual trial-and-error refinement of the results.
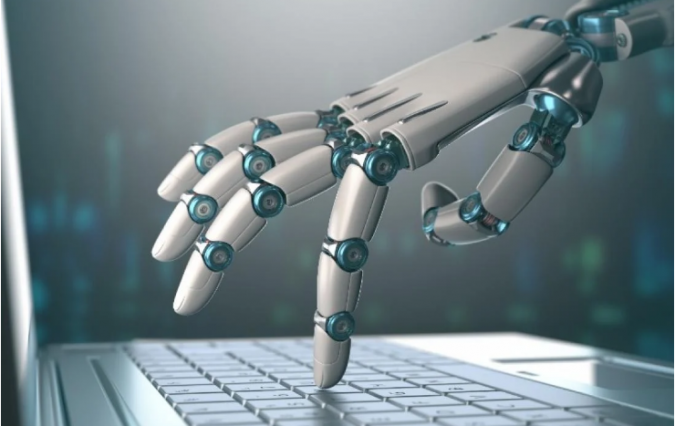
“To reduce human costs and resources, we have developed a robotic process automation (RPA) system that we apply to an existing Rietveld analysis program called RIETAN-FP,” says Ryo Tamura of the NIMS team. “By using our new procedure, with the help of machine learning, we have succeeded in performing Rietveld analysis automatically,” Tamura adds.
The automation can be run on a personal computer and can reduce human error as well as high-speed data analysis.
Tamura explains that the field of materials science already relies on numerous graphical user interface (GUI) applications to calculate a material’s properties, control experimental equipment, or analyze material data. He says that combining this new RPA and machine learning ability with these applications achieves a “closed loop” to automatically design and analyze materials with minimal human intervention.
The researchers verified the accuracy of their procedure by analyzing samples of powdered compounds whose crystal structures are already known. The ability to determine the structures from powdered samples is one of the great strengths of Rietveld’s analysis. It avoids the need to grow large single crystals, which can be extremely difficult to obtain for some materials.
“Automating Rietveld analysis brings a very powerful new tool into the entire field of materials science,” Tamura concludes.
The researchers are now working to further refine their procedure to make it suitable for more complex crystal structures. Another aim is to explore the use of their machine learning RPA strategy for more general applications in materials science.
The possibilities include numerous simulation methods used for calculating material properties, and also applications for controlling experimental equipment. The success achieved thus far with X-ray diffraction could just be the start for Rietveld robotics.