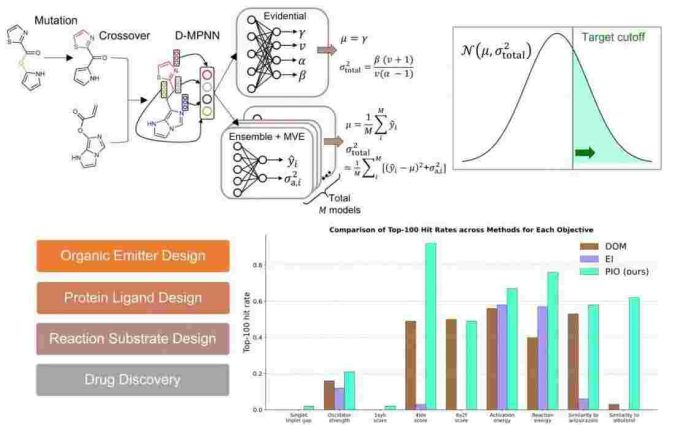
In a major step toward more reliable AI-assisted molecular design, researchers from National Taiwan University have demonstrated that incorporating uncertainty quantification (UQ) into graph neural network (GNN) models significantly improves both the efficiency and robustness of molecular optimization.
Their findings, recently published in Nature Communications, provide practical insights into how uncertainty-aware models can be integrated into computational-aided molecular design (CAMD) workflows to enhance decision-making in materials and drug discovery.
The research systematically investigates whether UQ-enhanced directed message passing neural networks (D-MPNNs) can effectively guide optimization across broad, open-ended chemical spaces, where the limitations of conventional machine learning models often become apparent.
These open domains are common in real-world applications but notoriously difficult to navigate due to the lack of reliable predictions for molecules that lie outside the training data distribution.
To address this, the team coupled D-MPNNs with genetic algorithms, enabling flexible and library-free molecular optimization. They evaluated multiple uncertainty-aware optimization strategies across 16 diverse benchmark tasks from the Tartarus and GuacaMol platforms.
These tasks reflect key challenges in organic electronics, reaction engineering, and drug development, including multi-objective scenarios that require balancing trade-offs between competing molecular properties.
Among the strategies tested, probabilistic improvement optimization (PIO) consistently delivered superior performance. By leveraging UQ to estimate the likelihood that a candidate molecule will meet design thresholds, PIO effectively avoids unreliable extrapolations and steers the search toward chemically promising regions.
“Chemical design often requires finding a balance between multiple competing objectives within vast and uncertain search spaces. By integrating uncertainty quantification into machine learning-guided optimization, we provide a principled way to navigate this complexity and enhance the reliability of AI-generated suggestions,” said Prof. Yi-Pei Li, the study’s corresponding author.
This work provides a robust foundation for uncertainty-aware molecular discovery, paving the way for more dependable and data-efficient AI in chemistry and materials science.