New technique greatly reduces the number of exposures necessary for “lensless imaging.”
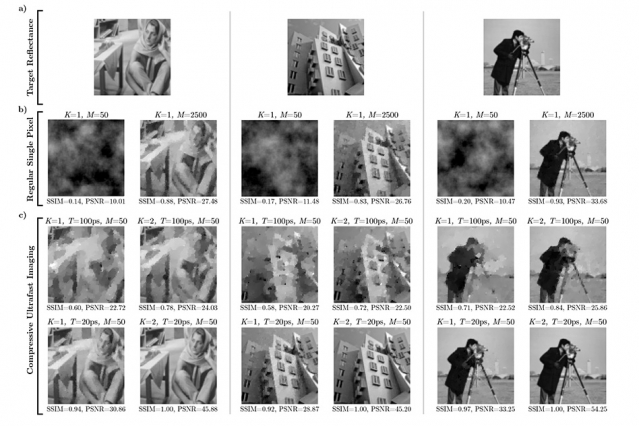
Courtesy of the researchers
CAMBRIDGE, Mass. — Compressed sensing is an exciting new computational technique for extracting large amounts of information from a signal. In one high-profile demonstration, for instance, researchers at Rice University built a camera that could produce 2-D images using only a single light sensor rather than the millions of light sensors found in a commodity camera.
But using compressed sensing for image acquisition is inefficient: That “single-pixel camera” needed thousands of exposures to produce a reasonably clear image. Reporting their results in the journal IEEE Transactions on Computational Imaging, researchers from the MIT Media Lab now describe a new technique that makes image acquisition using compressed sensing 50 times as efficient. In the case of the single-pixel camera, it could get the number of exposures down from thousands to dozens.
One intriguing aspect of compressed-sensing imaging systems is that, unlike conventional cameras, they don’t require lenses. That could make them useful in harsh environments or in applications that use wavelengths of light outside the visible spectrum. Getting rid of the lens opens new prospects for the design of imaging systems.
“Formerly, imaging required a lens, and the lens would map pixels in space to sensors in an array, with everything precisely structured and engineered,” says Guy Satat, a graduate student at the Media Lab and first author on the new paper. “With computational imaging, we began to ask: Is a lens necessary? Does the sensor have to be a structured array? How many pixels should the sensor have? Is a single pixel sufficient? These questions essentially break down the fundamental idea of what a camera is. The fact that only a single pixel is required and a lens is no longer necessary relaxes major design constraints, and enables the development of novel imaging systems. Using ultrafast sensing makes the measurement significantly more efficient.”
Recursive applications
One of Satat’s coauthors on the new paper is his thesis advisor, associate professor of media arts and sciences Ramesh Raskar. Like many projects from Raskar’s group, the new compressed-sensing technique depends on time-of-flight imaging, in which a short burst of light is projected into a scene, and ultrafast sensors measure how long the light takes to reflect back.
The technique uses time-of-flight imaging, but somewhat circularly, one of its potential applications is improving the performance of time-of-flight cameras. It could thus have implications for a number of other projects from Raskar’s group, such as a camera that can see around corners and visible-light imaging systems for medical diagnosis and vehicular navigation.
Many prototype systems from Raskar’s Camera Culture group at the Media Lab have used time-of-flight cameras called streak cameras, which are expensive and difficult to use: They capture only one row of image pixels at a time. But the past few years have seen the advent of commercial time-of-flight cameras called SPADs, for single-photon avalanche diodes.
Though not nearly as fast as streak cameras, SPADs are still fast enough for many time-of-flight applications, and they can capture a full 2-D image in a single exposure. Furthermore, their sensors are built using manufacturing techniques common in the computer chip industry, so they should be cost-effective to mass produce.
With SPADs, the electronics required to drive each sensor pixel take up so much space that the pixels end up far apart from each other on the sensor chip. In a conventional camera, this limits the resolution. But with compressed sensing, it actually increases it.
Getting some distance
The reason the single-pixel camera can make do with one light sensor is that the light that strikes it is patterned. One way to pattern light is to put a filter, kind of like a randomized black-and-white checkerboard, in front of the flash illuminating the scene. Another way is to bounce the returning light off of an array of tiny micromirrors, some of which are aimed at the light sensor and some of which aren’t.
The sensor makes only a single measurement — the cumulative intensity of the incoming light. But if it repeats the measurement enough times, and if the light has a different pattern each time, software can deduce the intensities of the light reflected from individual points in the scene.
The single-pixel camera was a media-friendly demonstration, but in fact, compressed sensing works better the more pixels the sensor has. And the farther apart the pixels are, the less redundancy there is in the measurements they make, much the way you see more of the visual scene before you if you take two steps to your right rather than one. And, of course, the more measurements the sensor performs, the higher the resolution of the reconstructed image.
Economies of scale
Time-of-flight imaging essentially turns one measurement — with one light pattern — into dozens of measurements, separated by trillionths of seconds. Moreover, each measurement corresponds with only a subset of pixels in the final image — those depicting objects at the same distance. That means there’s less information to decode in each measurement.
In their paper, Satat, Raskar, and Matthew Tancik, an MIT graduate student in electrical engineering and computer science, present a theoretical analysis of compressed sensing that uses time-of-flight information. Their analysis shows how efficiently the technique can extract information about a visual scene, at different resolutions and with different numbers of sensors and distances between them.
They also describe a procedure for computing light patterns that minimizes the number of exposures. And, using synthetic data, they compare the performance of their reconstruction algorithm to that of existing compressed-sensing algorithms. But in ongoing work, they are developing a prototype of the system so that they can test their algorithm on real data.