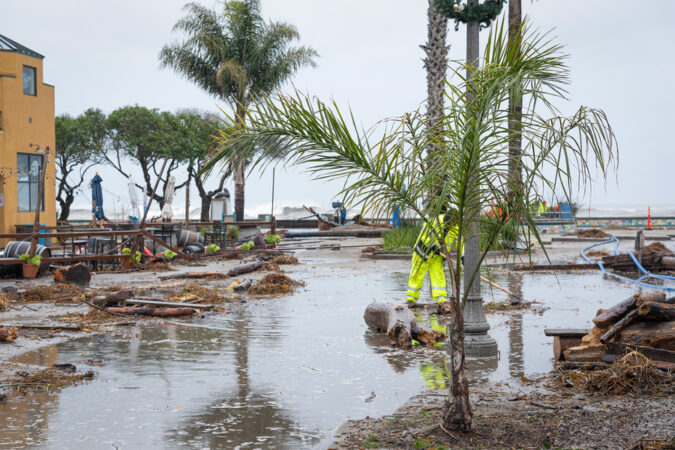
CAMBRIDGE, Mass. — To assess a community’s risk of extreme weather, policymakers rely first on global climate models that can be run decades, and even centuries, forward in time, but only at a coarse resolution. These models might be used to gauge, for instance, future climate conditions for the northeastern U.S., but not specifically for Boston.
To estimate Boston’s future risk of extreme weather such as flooding, policymakers can combine a coarse model’s large-scale predictions with a finer-resolution model, tuned to estimate how often Boston is likely to experience damaging floods as the climate warms. But this risk analysis is only as accurate as the predictions from that first, coarser climate model.
“If you get those wrong for large-scale environments, then you miss everything in terms of what extreme events will look like at smaller scales, such as over individual cities,” says Themistoklis Sapsis, the William I. Koch Professor and director of the Center for Ocean Engineering in MIT’s Department of Mechanical Engineering.
Sapsis and his colleagues have now developed a method to “correct” the predictions from coarse climate models. By combining machine learning with dynamical systems theory, the team’s approach “nudges” a climate model’s simulations into more realistic patterns over large scales. When paired with smaller-scale models to predict specific weather events such as tropical cyclones or floods, the team’s approach produced more accurate predictions for how often specific locations will experience those events over the next few decades, compared to predictions made without the correction scheme.
Sapsis says the new correction scheme is general in form and can be applied to any global climate model. Once corrected, the models can help to determine where and how often extreme weather will strike as global temperatures rise over the coming years.
“Climate change will have an effect on every aspect of human life, and every type of life on the planet, from biodiversity to food security to the economy,” Sapsis says. “If we have capabilities to know accurately how extreme weather will change, especially over specific locations, it can make a lot of difference in terms of preparation and doing the right engineering to come up with solutions. This is the method that can open the way to do that.”
The team’s results appear today in the Journal of Advances in Modeling Earth Systems. The study’s MIT co-authors include postdoc Benedikt Barthel Sorensen and Alexis-Tzianni Charalampopoulos SM ’19, PhD ’23, with Shixuan Zhang, Bryce Harrop, and Ruby Leung of the Pacific Northwest National Laboratory in Washington state.
Over the hood
Today’s large-scale climate models simulate weather features such as the average temperature, humidity, and precipitation around the world, on a grid-by-grid basis. Running simulations of these models takes enormous computing power, and in order to simulate how weather features will interact and evolve over periods of decades or longer, models average out features every 100 kilometers or so.
“It’s a very heavy computation requiring supercomputers,” Sapsis notes. “But these models still do not resolve very important processes like clouds or storms, which occur over smaller scales of a kilometer or less.”
To improve the resolution of these coarse climate models, scientists typically have gone under the hood to try and fix a model’s underlying dynamical equations, which describe how phenomena in the atmosphere and oceans should physically interact.
“People have tried to dissect into climate model codes that have been developed over the last 20 to 30 years, which is a nightmare, because you can lose a lot of stability in your simulation,” Sapsis explains. “What we’re doing is a completely different approach, in that we’re not trying to correct the equations but instead correct the model’s output.”
The team’s new approach takes a model’s output, or simulation, and overlays an algorithm that nudges the simulation toward something that more closely represents real-world conditions. The algorithm is based on a machine-learning scheme that takes in data, such as past information for temperature and humidity around the world, and learns associations within the data that represent fundamental dynamics among weather features. The algorithm then uses these learned associations to correct a model’s predictions.
“What we’re doing is trying to correct dynamics, as in how an extreme weather feature, such as the windspeeds during a Hurricane Sandy event, will look like in the coarse model, versus in reality,” Sapsis says. “The method learns dynamics, and dynamics are universal. Having the correct dynamics eventually leads to correct statistics, for example, frequency of rare extreme events.”
Climate correction
As a first test of their new approach, the team used the machine-learning scheme to correct simulations produced by the Energy Exascale Earth System Model (E3SM), a climate model run by the U.S. Department of Energy, that simulates climate patterns around the world at a resolution of 110 kilometers. The researchers used eight years of past data for temperature, humidity, and wind speed to train their new algorithm, which learned dynamical associations between the measured weather features and the E3SM model. They then ran the climate model forward in time for about 36 years and applied the trained algorithm to the model’s simulations. They found that the corrected version produced climate patterns that more closely matched real-world observations from the last 36 years, not used for training.
“We’re not talking about huge differences in absolute terms,” Sapsis says. “An extreme event in the uncorrected simulation might be 105 degrees Fahrenheit, versus 115 degrees with our corrections. But for humans experiencing this, that is a big difference.”
When the team then paired the corrected coarse model with a specific, finer-resolution model of tropical cyclones, they found the approach accurately reproduced the frequency of extreme storms in specific locations around the world.
“We now have a coarse model that can get you the right frequency of events, for the present climate. It’s much more improved,” Sapsis says. “Once we correct the dynamics, this is a relevant correction, even when you have a different average global temperature, and it can be used for understanding how forest fires, flooding events, and heat waves will look in a future climate. Our ongoing work is focusing on analyzing future climate scenarios.”
This work was supported, in part, by the U.S. Defense Advanced Research Projects Agency.